1. 项目背景
本项目通过对数据挖掘领域中的关联规则经典算法Apriori,运用关联规则对某大型超市超市的部分数据进行分析、挖掘,判定发现不同类商品之间的关联度,挖掘出商品中隐藏的实用价值,进而在实际销售运作中有效地避免这类错误,给超市提出适当的货架销售建议与货架摆放依据,利于增加超市的运营利润。
2. 功能组成
基于 Python 的大型超市商品销售关联度分析系统的主要功能包括:
3. Apriori关联挖掘算法
3.1 关联规则挖掘定义
关联规则挖掘是数据挖掘领域的热点,关联规则反映一个对象与其他对象之间的相互依赖关系,如果多个对象之间存在一定的关联关系,那么一个对象可以通过其他对象进行预测。大多数关联规则挖掘算法通常采用的一种策略是,将关联规则挖掘任务分解为如下两个主要的子任务:
- 频繁项集产生(Frequent Itemset Generation)其目标是发现满足最小支持度阈值的所有项集,这些项集称作频繁项集;
- 规则的产生(Rule Generation)其目标是从上一步发现的频繁项集中提取所有高置信度的规则,这些规则称作强规则。关联分析的目标发现频繁项集;由频繁项集产生强关联规则,这些规则必须大于或等于最小支持度和最小置信度。
关联分析的目标是:
- 发现频繁项集;
- 由频繁项集产生强关联规则,这些规则必须大于或等于最小支持度和最小置信度。
3.3 支持度
支持度表示该数据项在事务中出现的频度。 数据项集X的支持度support(X)是D中包含X的事务数量与D的总事务数量之比,如下公式所示:
关联规则X=>Y的支持度等于项集X∪Y的支持度,如下公式所示:
如果support(X)大于等于用户指定的最小支持度minsup,则称X为频繁项目集,否则称X为非频繁项目集。
3.4 置信度
置信度也称为可信度,规则 X=>Y
的置信度表示D中包含X的事务中有多大可能性也包含Y。表示的是这个规则确定性的强度,记作confidence(X=>Y)。通常,用户会根据自己的挖掘需要来指定最小置信度阈值,记为minconf。
如果数据项集X满足support(X) >=
minsup,则X是频繁数据项集。若规则X=>Y同时满足confidence(X=>Y)>=minconf,则称该规则为强关联规则,否则称为弱关联规则。一般由用户给定最小置信度阈值和最小支持度阈值。发现关联规则的任务就是从数据库中发现那些置信度、支持度大于等于给定最小阈值的强关联规则。
4. 基于 Python 的大型超市商品销售关联度分析系统
4.1 系统注册登录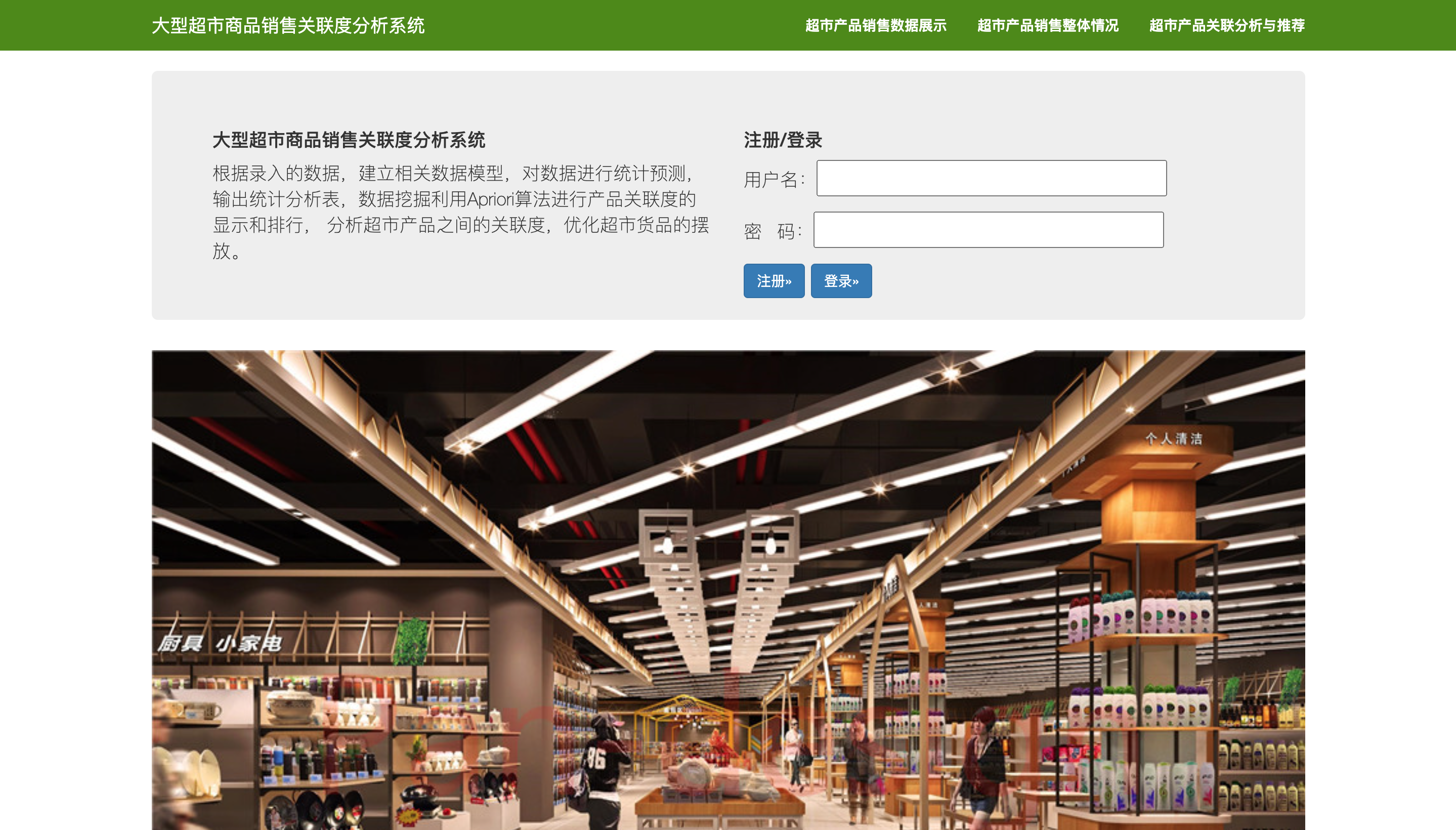
4.2 超市产品销售数据展示
4.3 超市产品销售整体情况可视化分析
针对超市近期销售数据进行统计分析和可视化:
@app.route(‘/product_analysis’)
def product_analysis():
“”“婴儿性别和年龄数据”“”
type_counts = type_df[‘Types’].value_counts().reset_index()
type_counts = type_counts.sort_values(by=‘index’)
# 用户一次购买商品数量分布
product_len_counts = {}
for pro in products:
jian_shu = ‘{}件’.format(len(pro))
if jian_shu not in product_len_counts:
product_len_counts[jian_shu] = 0
product_len_counts[jian_shu] += 1
product_len_counts = sorted(product_len_counts.items(), key=lambda x: x[1], reverse=True)
product_counts = {}
for pros in products:
for pro in pros:
if pro not in product_counts:
product_counts[pro] = 0
product_counts[pro] += 1
product_counts = sorted(product_counts.items(), key=lambda x: x[1], reverse=True)
return jsonify({
'类别': [g for g in type_counts['index'].values],
'商品个数': type_counts['Types'].values.tolist(),
'购买商品个数': [pc[0] for pc in product_len_counts],
'购买商品个数人次': [pc[1] for pc in product_len_counts],
'商品': [pc[0] for pc in product_counts],
'购买商品的人数': [pc[1] for pc in product_counts],
})
4.4 超市产品关联分析与推荐
@app.route(‘/clac_product_association//’)
def clac_product_association(min_support, min_threshold):
“”“计算产品之间的关联规则”“”
te = TransactionEncoder()
# 进行 one-hot 编码
te_ary = te.fit(products).transform(products)
df = pd.DataFrame(te_ary, columns=te.columns_)
# 利用 Apriori 找出频繁项集
freq = apriori(df, min_support=float(min_support), use_colnames=True)
# 计算关联规则
result = association_rules(freq, metric=“confidence”, min_threshold=float(min_threshold))
# 关联结果按照置信度或提升度高进行排序
result = result.sort_values(by=‘confidence’, ascending=False)
# 结果保存
result.to_csv(‘./results/product_association_min_support{}_{}.csv’.format(min_support, min_threshold),
encoding=‘utf8’, index=False)
results = result.to_dict(orient=‘records’)
for result in results:
result[‘antecedents’] = str(result[‘antecedents’])[10: -1] # 前因
result[‘consequents’] = str(result[‘consequents’])[10: -1] # 后果
return jsonify(results)
项目分享
项目分享:
https://gitee.com/asoonis/feed-neo